Collaboration takes aim at computational challenges in single-cell epigenomics
Single-cell epigenomic technologies promise fresh insight into the biology of human disease. But before this promise can be realized, one question must be answered: How do you draw meaningful conclusions from gargantuan single-cell epigenomic datasets? In collaboration with the Center, UC San Diego Assistant Professor Dr. Kyle Gaulton is capitalizing on his laboratory’s computational knowhow to answer this still-open question.
The question encapsulates a number of challenges associated with analyzing single-cell epigenomic data. How do you distinguish real cells from debris? Once identified, how do you assign cells to cell types? Are there other cell types that you don’t even know about? Are they actually different, or are they related to already known cell types? How do you know what’s meaningful?
“When we started working with the Center in 2017, there weren’t out-of-the-box solutions for analyzing single-cell epigenomic data,” Gaulton recalled.
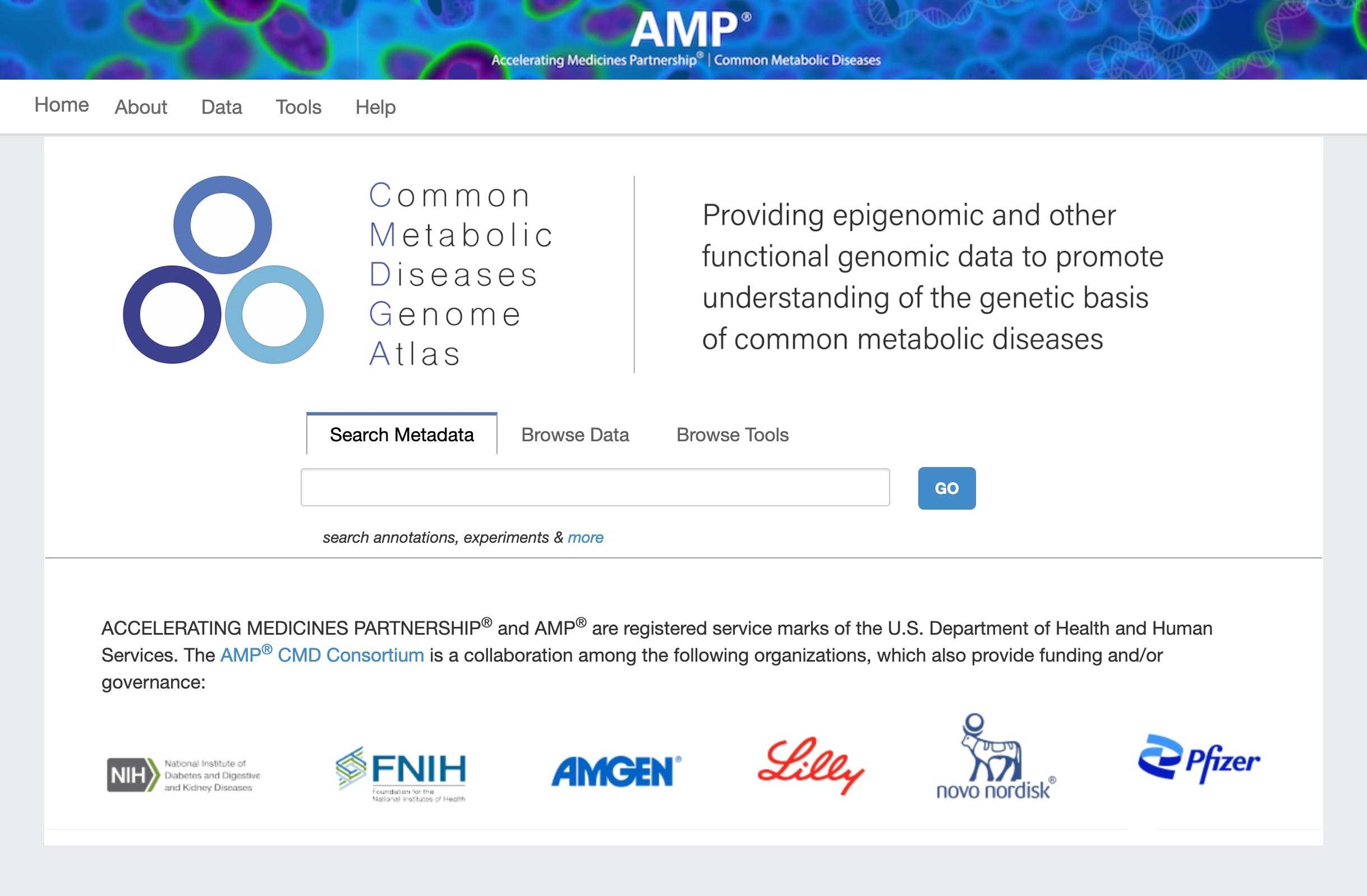
Gaulton’s ongoing partnership with the Center capitalizes the Center’s data-production capabilities to answer these questions within the context of type 1 and 2 diabetes. Collaborating with diabetes expert Dr. Maike Sander (UC San Diego), Gaulton’s laboratory helped chart a single-cell chromatin accessibility map of the human pancreas that revealed heterogeneous cellular states within distinct hormone-producing cell types involved in type 2 diabetes (see pp. X). In another study, the same group performed a largescale genome-wide association study (GWAS) with samples from type 1 diabetes patients and mapped a number of variants to candidate cis-regulatory elements in the pancreas and peripheral blood using single-cell ATAC-seq. Although both studies offered clues into the cellular origins of type 1 and 2 diabetes, Gaulton acknowledged that further validation is required to rule out any artifactual explanations to the data. After all, many studies have used singlecell technologies to define heterogeneity within the pancreas, for example, each with a different outcome.
Even with these maps under their belt, the question of what is meaningful lingers. Asked why too few singlecell studies agree with one another, Gaulton explained that such studies are often underpowered. One way to solve this problem is to sequence more deeply. Indeed, Gaulton and the Center did this in a single-nucleus ATAC-seq study of peripheral blood immune cells. “We only profiled 10 samples, yet we found thousands of variants that affected different cell types,” Gaulton said of the study.
As an alternative to deeper sequencing, multi-omics technologies like those being developed by the Center can be used to extract and align orthogonal information from each cell in a complex biological sample. These new technologies, however, are challenging to work with due to the vast amount of data they produce. In addition, the orthogonal data they produce can be difficult to integrate into a single map. “These are probably the biggest open challenges in single-cell epigenomics,” Gaulton said, before mentioning a grant he and the Center recently submitted to start tackling them.